Join Tina in a captivating conversation with Gloria Roque, Annotation Lead at Akrivia Health, as they unravel the intricacies of AI data annotation. From Gloria’s journey from biomedical science to AI annotation to the critical importance of logic over intuition in reducing bias, this episode delves deep into the collaborative nature of AI development. Gloria shares insights on addressing the gender divide in STEM education and empowering young women to pursue careers in AI.
Tina Marshall: Hello, my name’s Tina Marshall, and today I have Gloria Roque with me. Gloria is the Annotation Lead at Akrivia Health. Annotation works hand in hand with our AI scientists. Today, we’re going to be talking about how that all works, particularly as AI is such a big topic and very important to us. I want to touch on two points for this episode of the podcast; one being females in health tech. I enjoy speaking to Gloria for several different reasons. One of them is because she is one of the only females that I know who works in AI, so I want to touch on that with you. So thank you, Gloria, for coming and talking today.
Gloria: Thanks for having me.
Tina: But I also really wanted to dig into more of Gloria’s work and AI in general. It is such a big topic at the moment. Everybody’s throwing AI around, and for me, it’s one of those things where AI is such a big topic it is kind of like medical devices or pharma. It’s another speciality, it’s another function. We’ll dig into that. As I said, thanks Gloria, I appreciate having you here. I think it’d be really good to start by understanding a bit more about you and your background.
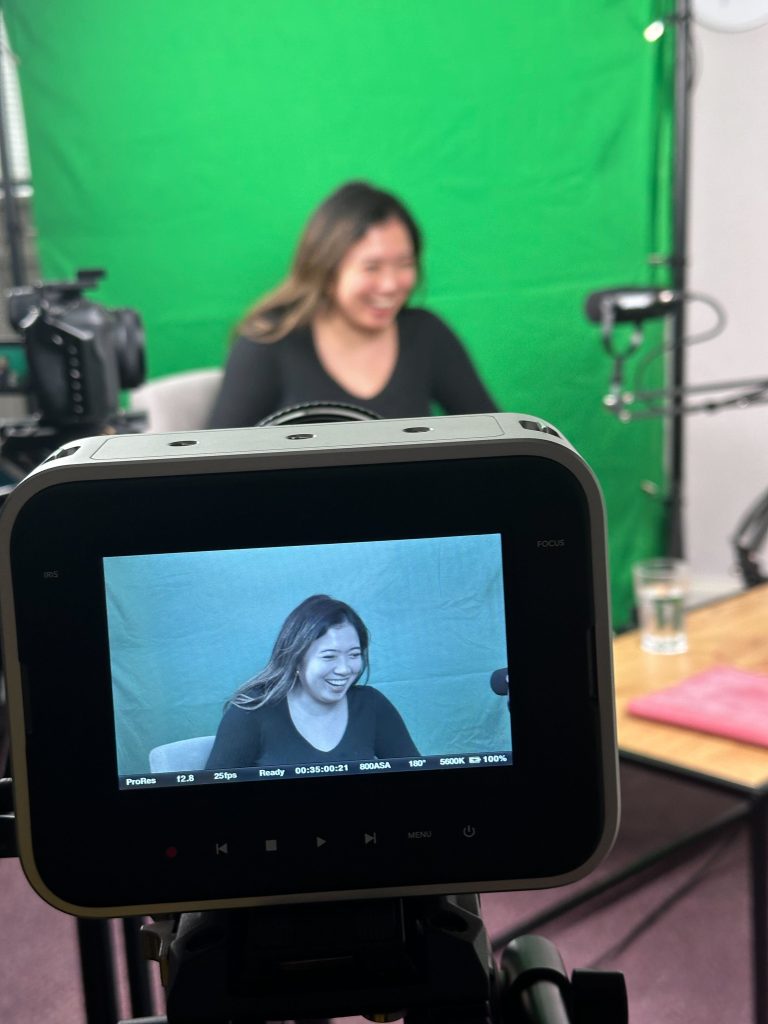
What led you to AI?
Gloria: That’s a really good question. I think what led me here was just really trying to figure out what I like and what I don’t like, and saying yes to opportunities. My background is actually in biomedical science, and initially, I worked in the hospital as a scientist in the cancer lab and I realised that, whilst it was a great job, I just didn’t want to be working in the hospital. I had this opportunity with a society social at Oxford University, and there, I met a couple of people from the Oxford AI Society. They asked me to be part of their committee. So I was just doing some small things like arranging social events for them, arranging hackathons. And that was a time when I started seeing the potential of AI and I figured I wanted to do my Master’s in Health Data Science. And after that, I got a job here in Akrivia. It wasn’t like it was, Akrivia was still starting then and I had the opportunity to shape my role and I knew I wanted to be in the AI team. It was just that I didn’t know which aspect of AI I wanted to be in. Now I’m in data annotations of AI.
Tina: So going back then, Gloria, what led you to biomedical science? Because it doesn’t seem as though it’s something that’s presented to women or young women. And it could be I’m much older than you. We’re not going to say how much, but even I remember. In September, in a Facebook group for parents, some parents had children who had just gone up to college, but they didn’t get the grades that they wanted at college to do the courses that they wanted to do, which were scientific engineering. So they were automatically put into a childcare course, which to me is genuinely crazy to think that that happens in this day and age, none of the boys who didn’t get their grades were put into childcare. It was just the girls. So for me, it’s shocking.
How was it presented to you? What led you to want to do that in the first place?
Gloria: Biomedical science? Well, it was mostly my parents. My mom is a nurse and my dad is a doctor, and I thought I’d like to be in that field. I thought at first I wanted to be a doctor, but I didn’t want to be in the hospital. And that’s how I figured it out, doing that lab work in the hospital. And then I just decided this is not what I want. I wanted something around health but not necessarily be in the hospital, and I was exploring my options for that. And then that’s when I came across AI. They were already talking about AI in the lab, AI machines, and so I just started exploring the different aspects of health.
Tina: Brilliant. And now you work in data annotation, that’s your thing?
What is data annotation and how does it relate to AI?
Gloria: I feel it’s not talked about because in AI I feel like it’s mostly the models that we know. Data annotations are part of model development and I’d like to separate model development into two parts, which is the data-centric aspect of it. So that’s where the data annotations, data labelling, and data curation sit in. So that’s where I sit. And then also you have the model-centric aspect of it; that’s what Tarso does. They’re AI scientists, so that’s the algorithms, the architecture, the models. Data annotation in its simplest terms is adding a label or a tag on a text so that you can elaborate on the context more. You do that all the time when you read, you highlight a word and then you add context to it. That’s what we do. In terms of AI data, annotation is the process in preparation for training the model. This is when you label the data so that you can train the model in the tasks that they need to do. And that’s very important because that’s the foundation at which the model learns. So that’s why I try to separate the model and the data side, but they come together.
Tina: Everything that you’ve just said for data annotation, I’m thinking, wow, you must have great attention to detail to be able to zero in on that data with a precise focus.
Gloria: Yeah. So talking about the strengths of annotation, I think you’re right, it’s attention to detail, but I guess it’s also collaboration. It’s a lot of collaboration because when you read a text, person A would interpret that differently from person B. It’s very subjective, and one of the challenges in annotation is the subjectiveness of the text. And you want, for the model to learn, you need high-quality consistent data. And that means it needs to be thought the way you approach annotation should be more logical rather than intuitive. Because I feel like most people who don’t do the annotations or data labelling would think that, oh yeah, data labelling. When you read a text, you know what it means. It’s so intuitive. And that’s what you want to avoid intuitiveness because that’s where you introduce bias, basically the intuition, that’s your mental shortcut on how you interpret things. And the model can’t learn that the model learns differently. It needs to be logical, it needs to be consistent, and it needs to be reproducible because when you think about your intuition, sometimes you can’t explain it. It’s just my intuition. You can’t really say that to the client, be like, yeah, that’s my intuition. No, it needs to be like, you need to be able to logically explain why you labelled this data like this.
Tina: So removing that intuition, focusing on the logic part of it, that helps to reduce bias in the AI model because there are lots of conversations at the moment about bias.
How can we reduce bias and how can we make sure that AI isn’t naturally biased because of how it’s being trained?
Gloria: Yes. I mean bias by its definition is when the model makes the wrong assumptions and it gives the wrong answers. And that’s because the training data it was given was not reflective of the real world. And that’s what we want to avoid, the bias.
“But it’s hard to avoid bias because bias is everywhere. Bias is in human language, the way we write things, and the way we interpret them is a form of bias, and that’s why it’s quite difficult to kind of reduce it.”
But recognising your own biases and recognise where they may arise because it does arise in the different stages of the AI pipeline. So if you recognise that bias might happen here.
Do people recognise their own bias?
Gloria: I guess it’s so difficult. You do recognise and you realise it when you collaborate and talk to other people and you’re just like, oh yeah, sometimes it’s so automatic. Intuition is so automatic, and you don’t realise that you’re being intuitive until someone calls you out and says, I don’t think it’s like this. It’s like this. Also, this is why it’s very important in annotation that you have proper documentation of rules and logic because sometimes you contradict yourself, and this is what I’m talking about, consistency. You should be consistent. Annotation is better done in a cross-team collaboration with our subject matter experts. So we have our clinical psychiatrists that we talk to for advice as well. And having just a well-rounded team with different perspectives avoids that unintentional bias.
How can we help young women move into AI?
Gloria: That’s a really good question. I think being aware of it at a very young stage, especially in school would help. And I’m just basing this on my experience and taking it as a great result. Maybe this is just in my school, the interest in physics and maths tends to be male-dominated, and physics and maths are kind of the requirements for working in AI.
Why is it at school then that there are just many boys doing physics?
Gloria: Honestly, I don’t know. I did psychology, but I didn’t want to be there. You tend to go with your friends or, as I found with physics A-Level, it didn’t really interest me because it was almost all boys in the class. There was only one girl there. And it was the opposite in psychology. There were only two guys.
Tina: Definitely a gender divide going on there. Women want to find out what’s going on in people’s heads, what makes you tick. And men just want to get things to tick.
Gloria: I guess it’s more because if you give them a choice, then kids usually choose where they’re more comfortable. Sometimes it’s not even the subject, it’s the presence of the people.
Tina: The friends and their teachers. I think that’s a really good point. I remember when I was at school, I had a choice of doing French and German. I was good at French, but I didn’t like the French teacher. I chose German. That wasn’t a clever move on my part.
Gloria: I mean, the same for me. I wanted to do history, but my friends chose drama, so I did drama. It was fun. But I wish I had chosen history. I don’t necessarily have the answer to how you break those barriers because those are the barriers that I experienced. And I think it is just more of, I guess collaborating, mixing people up. Collaboration is key.
Tina: Do you know Gloria, I genuinely have a feeling that just everything that you’ve said here will help young women see that working in AI is an option for them. Thank you very much, Gloria, for joining me today. I think it’s been really fun to speak to you and for everybody listening to this one; annotation and the AI modelling. It’s not one or the other. They need to work seamlessly together. So everything that I’ve heard through today with speaking to Gloria is around collaboration. As you’ll know, I have a keen interest in moving more women into STEM. And I think even just listening to Gloria and her experiences, just goes to show that we need to start at the beginning, start early. So this goes as a bit of a shout-out to the schools and the lecturers and the colleges.
For this episode of Precision Neuroscience Reimagined, and more: https://podcasters.spotify.com/pod/show/precision-neuroscience/episodes/Breaking-Barriers-Women-in-AI-e2glmg3 OR you can find the full episode on our YouTube channel: https://youtu.be/xz7dZo1OH2c